Crossing the Chasm
By Nitin Chaturvedi, Former Global Chief Digital and Technology Officer at KFC, ex-McKinsey, and Google
We hear about artificial intelligence (AI) everywhere. In podcasts, daily news headlines, and tweets of entrepreneurs. AI is being heralded as the technology that will bring a never-seen-before societal transformation. Both big technology companies and startups in Silicon Valley are rushing to realize this promise, investing billions of dollars in the process.
Yet, AI adoption in main street, though increasing, is not widespread or at scale. According to a recent Gartner study, only 9% of organizations they surveyed were AI-mature, defined as organizations that deploy AI widely across business units and processes[i]. A 2024 McKinsey survey reinforces this – only 11% of organizations are using Gen AI at scale[ii]. Hence, there exists a deep chasm today between the promise of AI and the realization of this promise in main street.
Why? It is not because main street leaders don’t appreciate AI’s transformative power. There are enough proof points of its potential. I believe there are three fundamental barriers that AI solution providers have to overcome to achieve adoption at scale at their target main street clients:
Model readiness
Despite rapid advancements, many market AI models haven’t matured to the point where they are ready for scale. Examples include:
- models that are accurate most of the time, but not all the time.
- models that lack robustness to handle big swings in input data.
- models with an unclear return on investment when you start scaling the proof-of-concept.
The above scenarios are highly problematic for main street companies looking to maximize returns and reduce risk, especially for customer-facing use cases.
Client readiness
Even if models are scale-ready, often, the target clients may not be ready to ingest these models at scale. Reasons vary by industry and company, but typical ones include – small AI allocations in the technology budget, lack of robust enterprise data to train models, gaps in AI-fluency of the existing talent base and an unwillingness to reimagine business workflows to be AI-first.
Fit to context
The final, and often overlooked, barrier is that AI solution providers do not adequately tailor their solutions and go-to-market strategies to align with the specific context of their clients. Understandably, this specific context is difficult to diagnose with an “outside-in” approach as it is determined by a complex and nuanced set of internal factors including the starting level of technology maturity, current priorities, internal ROI thresholds, and organization dynamics, among others. However, startups that can understand and adapt to the specific context can also get a significant leg up on the path to scale.
Below are six practical strategies to help AI solution providers overcome this last barrier. Although this is not an exhaustive list, these are the key barriers that need to be addressed. Since there is a wealth of articles and insights in the media and industry publications on solutions for the first two barriers, I will focus on the last in this article.
- Flyswatter or machine gun: The most basic check that AI solution providers should do to adapt to the specific client context is ask the question: “Does the client use case even need AI”? In many cases, such as business process automation, a less sophisticated and more cost-effective technology, like Robotic process automation may be good enough to meet the need. Another example is an AI personalization model, which can be valuable for retailers with variety of products in the average customer’s basket but not needed when customers add the same one or two products to their basket – think fries and a drink for a typical fast-food customer. In such cases, using an AI solution is like using a machine gun to kill a fly. It might get the job done, but a simple flyswatter would be much more appropriate.
- Stand on the big rocks: A typical CIO must keep the lights on for foundational technology, grow sales, drive innovation and reduce risk. Investments and mindshare are split across these, with a few key priorities on the CIO agenda, which are influenced by factors such as current maturity, strategy, resourcing, budgets, feasibility. AI initiatives are one contender within this massive agenda, typically within the innovation bucket. Given the uncertainties of its returns and the fact that many main street companies have more foundational problems to solve such as technology modernization, it can get pushed down the agenda quickly. Thus, ensuring their AI solution directly piggybacks off one of the CIO’s big three priorities significantly improve the chances for a startup to get on the path to scale.
- Connected models: Most solution providers bring point solutions that solve specific use cases such as knowledge management, coding productivity and customer support efficiency. Very few consider integrating these with other AI solutions to create a daisy chain that can transform how businesses are run. One example for a retailer would be connecting an advanced AI forecasting model with an inventory model to predict excess inventory, a pricing model to price this excess, a promotion model to create an offer and a loyalty model to prioritize customers to send this offer to. This type of cross-domain connections across multiple AI models can improve the returns vs. point AI implementations and improve the likelihood of passing the internal ROI threshold for scale.
- Soft trumps hard: The default go-to-for many AI solution providers is their superior product features and integration ease. While this is necessary, unfortunately it’s also table stakes. What is often overlooked is the soft factors needed to establish trust and credibility, the biggest ones being cross-functional orchestration and chemistry with the on-the-ground teams.
CIOs need to bring along their cross-functional CXO counterparts (e.g., the CMO, the COO) to ensure any AI product gets organizational mindshare. To enable this orchestration, solution providers need to first understand existing organizational relationship dynamics and then bridge any gaps through a simultaneous dialogue with each of the CXOs. In addition, startups need to build deep trust with the on-the-ground working teams from the first interaction, because often CIO’s will defer the final decision to them, given they are closest to the ground realities. Thus, thinking beyond the initial sale to address soft factors at the top and bottom of an organization can give startups the necessary support to achieve downstream scale.
- Fringes before core: Every solution provider wants more and larger customers to rapidly scale their revenues and reach unicorn status. Hence, when they try to break in, they often pick the bigger parts of a business e.g., large geographical regions or high revenue lines of business. However, these bigger parts of the business also have more wood to chop – more stakeholders to align, a deeper scrutiny of investments and a lower risk tolerance. In contrast, if a solution provider can pick a fringe part of the business, such as a small geography to prove their worth, their chances of further scaling the business can dramatically improve. The reality is that business unit leaders place far more trust in peer word-of-mouth endorsements than pitch decks. Therefore, a strong reputation established in a smaller (and easier) part of the business can significantly accelerate the downstream speed of propagation in the rest of the network. Conquer the fringes first and the core will follow.
- The ‘missing’ half of technology: Less than half of any technology’s adoption in an organization is determined by a winning AI product. The other half (or more) comes from a ready-to-go capability toolkit to operationalize this AI product without putting the existing company eco-system at risk. These include cost-effective model maintenance approaches, synthetic data generation capabilities for enterprise pockets with poor data, partnerships in international markets to implement the product, pricing models that can flex to low-cost countries and pre-built integrations with an organization’s back-end systems. Collectively, these capabilities mitigate downstream risk and investment, which is a critical precursor to scaling a product.
AI is unquestionably an exciting technology with immense potential. However, realizing this potential will require AI solution providers to develop a cost-effective and quick path to scale in main street companies. To cross the current chasm between promise and reality, AI solution providers must overcome three main barriers - Model readiness, Client readiness and Fit to context. Of these, the last barrier is the hardest to surmount, as it is shaped by complex, internal factors. Nonetheless, a thoughtful approach, leveraging the six tailored strategies outlined in this article, can significantly accelerate their path to success.
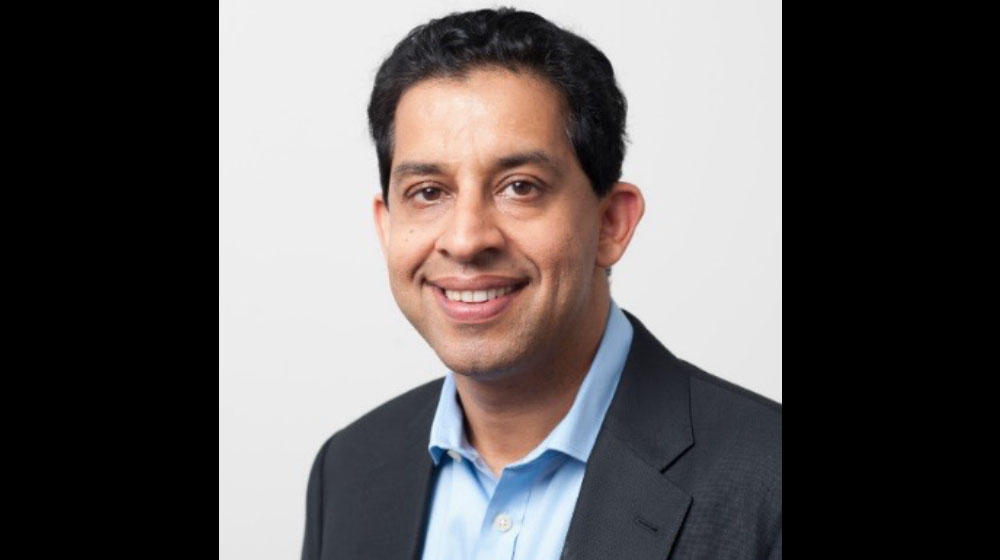
About the author
Nitin is a commercially oriented technologist with global leadership experience in strategy, digital transformation, and AI. He has driven growth and efficiency across industries, leveraging expertise from leading technology firms and consulting organizations. Nitin holds advanced degrees from Wharton, Purdue, and IIT and serves on multiple boards, including the North Dallas Food Bank and Evanta. He is a sought-after speaker at industry forums like the Economist Innovation @Work Summit.
By Nitin Chaturvedi, Former Global Chief Digital and Technology Officer at KFC, ex-McKinsey, and Google
We hear about artificial intelligence (AI) everywhere. In podcasts, daily news headlines, and tweets of entrepreneurs. AI is being heralded as the technology that will bring a never-seen-before societal transformation. Both big technology companies and startups in Silicon Valley are rushing to realize this promise, investing billions of dollars in the process.
Yet, AI adoption in main street, though increasing, is not widespread or at scale. According to a recent Gartner study, only 9% of organizations they surveyed were AI-mature, defined as organizations that deploy AI widely across business units and processes[i]. A 2024 McKinsey survey reinforces this – only 11% of organizations are using Gen AI at scale[ii]. Hence, there exists a deep chasm today between the promise of AI and the realization of this promise in main street.
Why? It is not because main street leaders don’t appreciate AI’s transformative power. There are enough proof points of its potential. I believe there are three fundamental barriers that AI solution providers have to overcome to achieve adoption at scale at their target main street clients:
Model readiness
Despite rapid advancements, many market AI models haven’t matured to the point where they are ready for scale. Examples include:
- models that are accurate most of the time, but not all the time.
- models that lack robustness to handle big swings in input data.
- models with an unclear return on investment when you start scaling the proof-of-concept.
The above scenarios are highly problematic for main street companies looking to maximize returns and reduce risk, especially for customer-facing use cases.
Client readiness
Even if models are scale-ready, often, the target clients may not be ready to ingest these models at scale. Reasons vary by industry and company, but typical ones include – small AI allocations in the technology budget, lack of robust enterprise data to train models, gaps in AI-fluency of the existing talent base and an unwillingness to reimagine business workflows to be AI-first.
Fit to context
The final, and often overlooked, barrier is that AI solution providers do not adequately tailor their solutions and go-to-market strategies to align with the specific context of their clients. Understandably, this specific context is difficult to diagnose with an “outside-in” approach as it is determined by a complex and nuanced set of internal factors including the starting level of technology maturity, current priorities, internal ROI thresholds, and organization dynamics, among others. However, startups that can understand and adapt to the specific context can also get a significant leg up on the path to scale.
Below are six practical strategies to help AI solution providers overcome this last barrier. Although this is not an exhaustive list, these are the key barriers that need to be addressed. Since there is a wealth of articles and insights in the media and industry publications on solutions for the first two barriers, I will focus on the last in this article.
- Flyswatter or machine gun: The most basic check that AI solution providers should do to adapt to the specific client context is ask the question: “Does the client use case even need AI”? In many cases, such as business process automation, a less sophisticated and more cost-effective technology, like Robotic process automation may be good enough to meet the need. Another example is an AI personalization model, which can be valuable for retailers with variety of products in the average customer’s basket but not needed when customers add the same one or two products to their basket – think fries and a drink for a typical fast-food customer. In such cases, using an AI solution is like using a machine gun to kill a fly. It might get the job done, but a simple flyswatter would be much more appropriate.
- Stand on the big rocks: A typical CIO must keep the lights on for foundational technology, grow sales, drive innovation and reduce risk. Investments and mindshare are split across these, with a few key priorities on the CIO agenda, which are influenced by factors such as current maturity, strategy, resourcing, budgets, feasibility. AI initiatives are one contender within this massive agenda, typically within the innovation bucket. Given the uncertainties of its returns and the fact that many main street companies have more foundational problems to solve such as technology modernization, it can get pushed down the agenda quickly. Thus, ensuring their AI solution directly piggybacks off one of the CIO’s big three priorities significantly improve the chances for a startup to get on the path to scale.
- Connected models: Most solution providers bring point solutions that solve specific use cases such as knowledge management, coding productivity and customer support efficiency. Very few consider integrating these with other AI solutions to create a daisy chain that can transform how businesses are run. One example for a retailer would be connecting an advanced AI forecasting model with an inventory model to predict excess inventory, a pricing model to price this excess, a promotion model to create an offer and a loyalty model to prioritize customers to send this offer to. This type of cross-domain connections across multiple AI models can improve the returns vs. point AI implementations and improve the likelihood of passing the internal ROI threshold for scale.
- Soft trumps hard: The default go-to-for many AI solution providers is their superior product features and integration ease. While this is necessary, unfortunately it’s also table stakes. What is often overlooked is the soft factors needed to establish trust and credibility, the biggest ones being cross-functional orchestration and chemistry with the on-the-ground teams.
CIOs need to bring along their cross-functional CXO counterparts (e.g., the CMO, the COO) to ensure any AI product gets organizational mindshare. To enable this orchestration, solution providers need to first understand existing organizational relationship dynamics and then bridge any gaps through a simultaneous dialogue with each of the CXOs. In addition, startups need to build deep trust with the on-the-ground working teams from the first interaction, because often CIO’s will defer the final decision to them, given they are closest to the ground realities. Thus, thinking beyond the initial sale to address soft factors at the top and bottom of an organization can give startups the necessary support to achieve downstream scale.
- Fringes before core: Every solution provider wants more and larger customers to rapidly scale their revenues and reach unicorn status. Hence, when they try to break in, they often pick the bigger parts of a business e.g., large geographical regions or high revenue lines of business. However, these bigger parts of the business also have more wood to chop – more stakeholders to align, a deeper scrutiny of investments and a lower risk tolerance. In contrast, if a solution provider can pick a fringe part of the business, such as a small geography to prove their worth, their chances of further scaling the business can dramatically improve. The reality is that business unit leaders place far more trust in peer word-of-mouth endorsements than pitch decks. Therefore, a strong reputation established in a smaller (and easier) part of the business can significantly accelerate the downstream speed of propagation in the rest of the network. Conquer the fringes first and the core will follow.
- The ‘missing’ half of technology: Less than half of any technology’s adoption in an organization is determined by a winning AI product. The other half (or more) comes from a ready-to-go capability toolkit to operationalize this AI product without putting the existing company eco-system at risk. These include cost-effective model maintenance approaches, synthetic data generation capabilities for enterprise pockets with poor data, partnerships in international markets to implement the product, pricing models that can flex to low-cost countries and pre-built integrations with an organization’s back-end systems. Collectively, these capabilities mitigate downstream risk and investment, which is a critical precursor to scaling a product.
AI is unquestionably an exciting technology with immense potential. However, realizing this potential will require AI solution providers to develop a cost-effective and quick path to scale in main street companies. To cross the current chasm between promise and reality, AI solution providers must overcome three main barriers - Model readiness, Client readiness and Fit to context. Of these, the last barrier is the hardest to surmount, as it is shaped by complex, internal factors. Nonetheless, a thoughtful approach, leveraging the six tailored strategies outlined in this article, can significantly accelerate their path to success.